Key Takeaways
- Item Response Theory (IRT) measures individual abilities and item difficulty through responses, enhancing test reliability and validity
- IRT is used in educational, psychological, and cognitive assessments to improve testing instruments and compare different populations
- IRT offers detailed insights for better assessments but also has practical limitations to consider
Item response theory (IRT) is a well-established statistical technique used to measure individual differences in abilities and attitudes. It is a powerful tool that allows researchers to elicit valid and reliable results that are independent of the test taker population. IRT is used in the assessment of educational, psychological and cognitive abilities, based on a respondent's responses to a set of items or questions.
This article will take an in-depth look at the fundamentals of item response theory. How IRT works will be explained, as well as its mechanisms, assumptions and implications. Additionally, the practical application of IRT will be discussed, as will potential benefits and drawbacks.
What is item response theory?
Item response theory (IRT) is an important tool in the world of psychometrics. IRT is a powerful and flexible statistical model used to measure an individual’s characteristics or abilities. It is a powerful method to assess a person’s performance on a given scale, such as aptitude tests, educational tests, and psychological tests. In other words, IRT is used to measure and study how a person answers a particular set of questions or items.
With the help of item response theory, researchers can better understand how people respond to particular items. This type of theory allows researchers to identify what an individual does well and what he or she may lack, as well as determining how difficult a particular set of items is for an individual. This knowledge can be used to develop more reliable, valid, and useful instruments for testing and assessment. Furthermore, IRT allows researchers to identify the skill level of a person, the difficulty of the items in the instrument, and even the inherent strengths and weaknesses of an individual.
In addition to this, item response theory allows users to evaluate how scores are derived from different individuals, as well as identify any potential areas of need. It is also a helpful tool in comparing the performance of different populations. Overall, IRT is an important tool in understanding individuals’ responses to different items. It provides a reasonable and reliable way to measure an individual’s performance on a given instrument, identify any potential areas of need, and compare scores between different populations.
Where is item response theory used?
Item response theory (IRT) is an important tool that is widely used in a variety of fields such as psychometrics, health sciences, educational psychology, and market research. It is an increasingly popular method for analyzing responses to items on tests, surveys, and other types of assessments. IRT is typically used to identify the best items to include in a test or survey and to build models that estimate the individual's performance or proficiency.
In educational assessment, IRT helps to identify student mastery or proficiency levels while also assisting in the development of new items or tests. By making the detailed analysis of data on student responses to various items, IRT helps educators and textbook developers understand what parts of the material are easiest or hardest for individual students. This helps educators make informed decisions about how to create more effective curricula and teaching methods.
In the field of psychometrics, IRT is used to measure a person’s mental ability, likes, dislikes, beliefs, and more. The analysis of items in terms of difficulty and individual responses can help researchers identify correlations between different items and allow for more in-depth analyses of the relationships between items.
IRT is also being increasingly used in healthcare research to study the effect of interventions for a variety of patient populations. In these studies, IRT helps researchers identify the factors that influence patient compliance with the intervention and better target treatments to those most receptive and compliant with their care.

(Source: Manchester 1824)
Hypothetical Item Characteristic Curves (ICCs), showing the relationship between the level of an unobserved trait and the probability of endorsing a binary questionnaire item, for three items. ICCs are a useful graphical way of understanding the relationships between item responses and participants’ underlying characteristics, or traits.
In conclusion, item response theory is an invaluable tool for a range of fields. By providing detailed data on the way people answer items on tests and surveys, IRT helps researchers, educators, and healthcare professionals alike better understand individual performance and hone in on tailored interventions and curricula.
How does item response theory help?
Item response theory (IRT) is a powerful tool in assessment and evaluation that provides an array of benefits to educational organizations, especially in the field of psychometrics. IRT can provide insights into how respondents are responding to questions, allowing for better test design and interpretation of results. It allows for more accurate and meaningful comparison of test results between different groups, identifying areas where certain parts of the test are better suited for one group than for another. IRT can also be used to identify scoring thresholds, providing guidance around how to approach each test question.
The use of IRT can support educators in a variety of ways. It helps reduce the complexity of tests, making them easier to design and implement, while also providing feedback on the efficacy of a test. IRT also acts as an unbiased instrument, providing insight into where areas may need to be improved or streamlined. With improved test design and interpretation of results, educators have greater clarity in creating learning goals and assessing performance.
The use of IRT has the potential to greatly enhance the educational experience for students, providing targeted and tailored learning experiences that are tailored to their individual needs. This helps create a more meaningful and personalized learning experience, which in turn can lead to improved performance and better outcomes for learners.
Decoding item response theory structure
Item Response Theory (IRT) is a powerful statistical framework used to develop, evaluate, and score assessments. The basic idea behind IRT is to understand how different test items (e.g., questions) function based on the responses of test-takers. The ultimate goal of IRT is to measure an individual's ability or proficiency in a given domain with as much accuracy as possible.
IRT models are based on the assumption that the probability of answering an item correctly depends on two main factors: the ability of the test-taker and the difficulty of the item. However, IRT models take this idea further by considering how item characteristics, such as the item's discrimination, can affect the probability of answering an item correctly.
The discrimination parameter of an item reflects how well the item discriminates between individuals who have a high level of ability and those who have a low level of ability. This means that an item with high discrimination will provide more information about the ability level of the test-taker than an item with low discrimination. In other words, an item with high discrimination will differentiate more effectively between those who know the material well and those who do not.
IRT models also allow for the estimation of item parameters such as item difficulty, discrimination, and guessing. Item difficulty refers to the level of ability required to have a 50% chance of answering an item correctly. Guessing refers to the probability of answering an item correctly by chance, without actually knowing the answer.
One of the most commonly used IRT models is the 3-parameter logistic (3PL) model, which includes item difficulty, discrimination, and guessing parameters. The 3PL model assumes that the relationship between the probability of answering an item correctly and a test-taker's ability is logistically shaped.
The estimation of item parameters and test-taker ability levels is usually done using maximum likelihood estimation (MLE). This involves finding the values of the model parameters that are most likely to have generated the observed data.
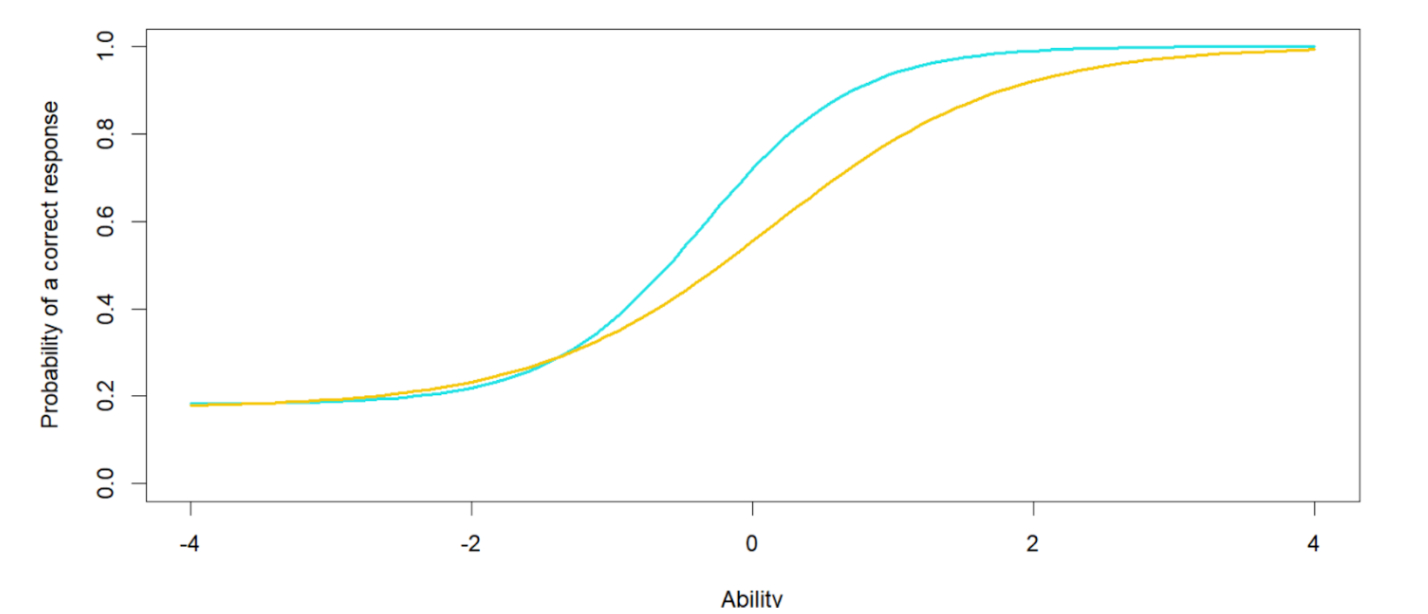
(Source: Mindfish)
The graph above shows us two different questions. The yellow question is harder, as it requires a higher ability level to have a 50% chance of a correct answer. The blue question is easier, and it also has a greater slope (discrimination) value—it provides more information about how much ability the respondent has.
The IRT models are particularly useful because they provide a range of information about individual items and test-takers. For instance, they can identify items that are too easy or too difficult, which can help to improve the quality of the assessment. They can also provide information about how well the items measure the underlying construct or ability being assessed.
Furthermore, IRT models can be used to create different scoring systems, which can make test scores more meaningful and useful for test-takers and educators. For example, test scores can be reported on a common scale, such as a 0 to 100 scale, even if the test consists of items with different levels of difficulty.
How item response theory works in digital SAT?
The 3-parameter logistic (3PL) model is a type of Item Response Theory (IRT) model that is commonly used in educational and psychological assessments, especially in SAT and ACT. With SAT going digital, the 3PL model is designed to estimate the probability of a test-taker answering an item correctly based on three parameters: item difficulty, discrimination, and guessing.
- Item difficulty: Item difficulty is the level of ability or proficiency required to have a 50% chance of answering the item correctly. In other words, item difficulty reflects how hard or easy the item is. For example, an item that requires a high level of ability or proficiency to answer correctly would be considered a difficult item, while an item that can be answered correctly by most test-takers would be considered an easy item.
- Discrimination: Discrimination is the degree to which an item distinguishes between test-takers who have high ability or proficiency levels and those who have low ability or proficiency levels. In other words, discrimination reflects how well an item can differentiate between test-takers who know the material well and those who do not. An item with high discrimination will provide more information about a test-taker's ability or proficiency level than an item with low discrimination.
- Guessing: Guessing is the probability of a test-taker answering an item correctly by chance, without actually knowing the answer. In multiple-choice items, guessing is the probability of choosing the correct answer among the available alternatives. Guessing is a crucial parameter to consider when developing assessments because it can affect the accuracy of the test scores.
The 3PL model assumes that the relationship between the probability of a test-taker answering an item correctly and the three parameters (item difficulty, discrimination, and guessing) is logistically shaped. This means that the probability of answering the item correctly increases as the test-taker's ability or proficiency level increases, but the rate at which the probability increases depends on the item's parameters.
The 3PL model is usually estimated using maximum likelihood estimation (MLE), which involves finding the values of the parameters that are most likely to have generated the observed data. The estimation process involves fitting the model to the data and adjusting the parameters until the model fits the data as closely as possible.
Once the 3PL model is estimated, it can be used to estimate the ability or proficiency levels of test-takers based on their responses to the items. The estimated ability levels can then be used to create scores or to make decisions about the test-takers' knowledge or skills.
The formula for the 3PL model is as follows:
P(X_i=1) = c_i + (1-c_i) / [1 + exp(-D_i(a-b))]
where:
- P(X_i=1) is the probability that the test-taker will answer item i correctly
- c_i is the guessing parameter for item i, which represents the probability of a test-taker answering the item correctly by guessing, without actually knowing the answer
- D_i is the discrimination parameter for item i, which reflects how well the item can distinguish between test-takers who have high levels of ability and those who have low levels of ability
- a is the test-taker's ability level on the latent trait being measured by the test (often denoted as theta or θ)
- b is the item difficulty parameter for item i, which reflects how difficult the item is for test-takers at the average level of ability
The logistic function 1/[1+exp(-D_i(a-b))] is used to transform the difference between the test-taker's ability level and the item difficulty into a probability. The guessing parameter c_i is included to account for the possibility that some test-takers may answer the item correctly by guessing.
By estimating the item difficulty, discrimination, and guessing parameters for each item on a test, and the ability level of each test-taker, the 3PL model can be used to estimate the probability of a test-taker answering each item correctly based on their ability level, and to estimate the test-taker's overall ability level on the latent trait being measured by the test.
Examples of a 3PL model:
Let’s look at an example with the above formula in place. For instance, we have a math test with 10 multiple-choice questions, each with four answer choices labeled A, B, C, and D. We administer this test to 100 students and want to use the 3PL IRT model to estimate each student's ability level.
For each question i, we have estimated the following parameters:
- Difficulty parameter: D_i
- Discrimination parameter: b_i
- Guessing parameter: c_i
We'll use the following values for one of the questions:
- D_i = -1.2
- b_i = 1.8
- c_i = 0.25
Now, let's say we want to estimate the probability of a student with ability level a_i answering this particular question correctly.
Using the 3PL IRT model formula, we have:
P(X_i=1) = c_i + (1-c_i) / [1 + exp(-D_i(a_i-b_i))]
Plugging in our example values, we get:
P(X_i=1) = 0.25 + (1-0.25) / [1 + exp(-(-1.2)(a_i-1.8))]
Simplifying this expression, we get:
P(X_i=1) = 0.25 + 0.75 / (1 + exp(1.2a_i - 2.16))
This formula gives us the estimated probability of a student with ability level a_i getting this particular question right. We can use this formula for all 10 questions on the test and for all 100 students who took the test to estimate their ability levels.
Let’s see another example with a different equation used in the IRT 3PL model.
We have a multiple-choice test with four options for each question. We will use the 3PL model to estimate the probability of a test-taker answering a particular item correctly.
We will start with a hypothetical item that measures a student's understanding of algebra:
Q: What is the value of x in the equation 3x + 5 = 14? a) 2 b) 3 c) 4 d) 5
The first step in applying the 3PL model is to estimate the item difficulty. We can estimate this value by analyzing the responses of a large group of test-takers who have taken the test. Suppose that 60% of the test-takers answered this question correctly. This suggests that the item is relatively easy, and we can estimate the difficulty parameter at -1.0 on the logit scale. The logit scale is a logarithmic transformation that converts the probability of a correct answer into a continuous scale.
Next, we estimate the discrimination parameter. This parameter reflects how well the item can distinguish between test-takers who have high levels of ability and those who have low levels of ability. One way to estimate the discrimination parameter is to compare the performance of test-takers who scored high on the test to those who scored low. Suppose that the item was answered correctly by 80% of high-scoring test-takers and by only 20% of low-scoring test-takers. This suggests that the item has a high discrimination parameter of 2.0.
Finally, we estimate the guessing parameter. This parameter reflects the probability of a test-taker answering the item correctly by guessing, without actually knowing the answer. Since this item has four options, the probability of guessing correctly is 0.25. Therefore, we estimate the guessing parameter at 1.386 (which is the natural logarithm of 0.25).
With these three parameters estimated, we can use the 3PL model to estimate the probability of a test-taker answering this item correctly based on their ability level. For example, suppose we have two test-takers, one with an estimated ability of -1.5 on the logit scale and another with an estimated ability of 1.5. We can use the 3PL model to estimate the probability of each test-taker answering this item correctly:
- Test-taker 1: p(correct) = e^(1.52 - 1.0) / (1 + e^(1.52 - 1.0) + e^(1.386)) p(correct) = 0.69 or 69%
- Test-taker 2: p(correct) = e^(1.52 - 1.0) / (1 + e^(1.52 - 1.0) + e^(1.386)) p(correct) = 0.98 or 98%
In this example, the 3PL model estimates that Test-taker 1 has a 69% chance of answering the item correctly, while Test-taker 2 has a 98% chance of answering the item correctly. This shows how the 3PL model can provide valuable information about a test-taker's ability level and the quality of test items.
Benefits of item response theory
Item Response theory (IRT) is a powerful tool for assessing test performance and determining proficiency levels. This comprehensive methodology has several practical benefits that can be used in exams and assessment systems.
- The first advantage is its robustness. IRT enables the analyses of items that can yield accurate, reliable results, even with items of varying difficulties or with a large number of examinees. This is incredibly beneficial as it allows examiners to gain accurate insights into the skills of their students.
- Second, IRT provides a powerful context in which to draw inferences. By using it, examiners can extrapolate information regarding the relative difficulty of different items, identify areas of strength or weakness in examinees, and better manage the challenges of constructing exams.
- Third, IRT is scalable. It is capable of producing results at both a group and individual level and its flexibility remarkably benefits practitioners who may be dealing with a diverse set of subject areas or changing exam formats.
- Fourth, IRT is a time-efficient method. Its ability to provide automated scoring for multiple levels of assessment can help to streamline the process of measuring student performance, allowing examiners to gain insight quickly.
- Finally, IRT is an invaluable aid in the development and improvement of exam items. Through its analysis of examinees’ responses, IRT can provide invaluable feedback which instructors can use to generate higher quality items that are more reflective of their intended objectives.
The benefits of utilizing IRT are manifold. Whether it be in the area of assessment, analysis, scaling, or item development, Item Response Theory is an invaluable tool for practitioners and students alike.
Drawbacks of item response theory
Item Response Theory (IRT) is a powerful tool that has helped immensely in the field of psychological testing and measurement, especially in terms of quantifying responses from test-takers. However, as with any model, there are certain drawbacks to IRT that should be considered.
- Firstly, IRT requires a relatively large sample size in order for it to be effective, and even with a sizable sample size, there is always the issue of extrapolating meaningful results for an entire population. This can become especially problematic when dealing with rare events or cases. Additionally, IRT does not allow for a granular-level of analysis, meaning certain questions or tests may not be able to be answered because of poor item design or poor administration of the test. This in turn can lead to a decrease in the predictive validity of the model or even erroneous results.
- In addition, there might also be questions as to how a model like IRT actually measures intelligence or other such mental states. While it can answer difficult questions, it is not entirely capable of doing so with any degree of accuracy. Additionally, there is considerable debate over the order of items in a given test, meaning that certain tests might yield different results depending on where the items are placed.
- Finally, IRT requires considerable expertise to create and use correctly, which add to its costs of implementation. While this is not a complete downfall, it can pose a challenge for those who don't know the theory or don't want to take the time to use it correctly.
Overall, while IRT has proven to be an invaluable asset to the testing landscape, it is not without its drawbacks, including the need for a large sample size, lack of detail analysis, and the need for expert knowledge. Knowing these considerations is essential in order to use IRT effectively.
Conclusion
In conclusion, Item Response Theory (IRT) is a valuable tool for designing and scoring tests or assessments, particularly those which include difficult or complex items. Its insights can be applied to both the development of tests and the analysis of results derived from the tests. Its models, based on probabilities and probabilities; as well as its capability to derive values of item difficulty and item-test correlation are some of the advantages that IRT offers. Furthermore, IRT can be used to detect and identify cheating on tests, given its ability to uncover anomalous response patterns.
It is clear that IRT is invaluable in terms of designing, administering, and interpreting the results of high-stakes assessments. As such, it’s important for instructors, curriculum designers, and test developers alike to understand the fundamentals of IRT and its associated concepts in order to benefit from its full potential. Understanding IRT can improve both the quality of tests and the subsequent interpretations of the test results. It is also crucial for educators to understand the impact of cheating and how to identify it using IRT. Overall, IRT provides a valuable approach to designing and evaluating tests, thus making it an invaluable asset for educators and researchers alike.
If you're looking to offer Digital SAT practice questions and assessments with the adaptive logic, then choose EdisonOS, the right platform that meets your organisation's needs.